
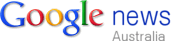
Mathematical model
SARS-CoV-2 virus infects and replicates in epithelial cells of the upper and lower respiratory tract18. We model this by developing a two patch within-host model, where the patches are the two respiratory tracts which are linked through virus migration between patches, or viral shedding. Both respiratory tract patches assume interactions between uninfected epithelial cells, \(T_j\); infected epithelial cells, \(I_j\); and virus homing in tract j, \(V_j\) at time t. Here, \(j=\\), with u describing the URT patch and l describing the LRT patch. Target cells in each patch get infected at rates \(\beta _j\) and infected cells produce new virions at rates \(p_j\). Infected cells die at rates \(\delta _j\) and virus particles are cleared at a linear rate \(c_u\) in the upper respiratory tract and in a density dependent manner \(c_l V_l/(V_l + K)\) in the lower respiratory tract, where K is the viral load in the LRT where the clearance is half maximal. The two patches are linked via the virus populations, with a proportion \(k_u\) of \(V_u\) migrating from URT to LRT and \(k_l\) of \(V_l\) migrating from LRT to URT. The model describing these interactions (see Fig. 1) is given by
$$\begin \begin \frac =&-\beta _u T_uV_u,\\ \frac =\,&\beta _u T_uV_u,- \delta _u I_u, \\ \frac =\,&p_u I_u - c_uV_u + k_l V_l, \\ \frac =\,&-\beta _l T_l V_l,\\ \frac =\,&\beta _lT_l V_l- \delta _l I_l, \\ \frac =\,&p_l I_l - c_l \fracV_l + k_u V_u.\\ \end \end$$
(1)
We model the initial conditions of the system Eq. (1) as follows. We assume that all epithelial cells in the URT and LRT patches are susceptible to virus infection. When infection occurs, it results in a small initial virus inoculum which homes in the URT alone. Under these assumptions, system Eq. (1) is subject to initial conditions
$$\begin \begin T_u(0)&= T_u^0, \ I_u(0) = 0, \ V_u(0) = V_0, \\ T_l(0)&= T_l^0, \ I_l(0) = 0, \ V_l(0) = 0, \end \end$$
(2)
where \(V_0\) is the viral inoculum. We aim to determine the dynamics of system Eq. (1) over time for model parameters that explain URT and LRT tract data in a single patient (patient A) and in the population data (all nine patients) from18.
Parameter estimation
Patient data
In January 2020, nine patients tested positive for COVID-19 in a single-source outbreak in Bavaria, Germany19. Early detection allowed for rapid contact tracing, testing, and monitoring of the affected community: young healthy professionals in their mid-thirties. A followup study published time series for the post symptoms virus data isolated from oral-and nasopharyngeal throat swabs (in copies per swabs) and from sputum samples (in RNA copies per mL) for the same patient population over their entire course of disease. The patients’ throat swabs and sputum data (Fig. 2 of 18) were obtained through personal communication with the authors. Since we know the incubation period for each patient19 (see Table 1), we assume time zero in our study to be the day of infection for the patients in Ref.18.
Identifiability analysis
Using the URT and LRT viral load data, we aim to determine the unknown parameters \(p = \\) of the within-host model Eq. (1). Before attempting to estimate the within-host model parameters using noisy laboratory data, it is crucial to analyze whether the model is structurally identifiable. Specifically, we need to know if the within-host model Eq. (1) is structured to reveal its parameters from upper and lower viral load observations. We approach this problem in an ideal setting where we assume that the observations are known for every \(t>0\) and they are not contaminated with any noise. This analysis is called structural identifiability27.
The observed data in Wolfel et al.18 is modeled in the within-host model Eq. (1) by variables \(V_u\) and \(V_l\), which account for the upper and lower respiratory tract viral titers. We denote these observed variable as
$$\begin y_1(t) = V_u(t) \quad \text \quad y_2(t) = V_l(t). \end$$
First, we give the definition of structural identifiability in terms of the observed variables \(y_1(t)\) and \(y_2(t)\)27,28,29,30,31.
Definition 1
Let \(p\) and \(q\) be the two distinct vectors of within-host model Eq. (1) parameters. We say that the within-host model is structurally (globally) identifiable if and only if
$$\begin y_1(t,p) = y_1(t,q) \quad \text \quad y_2(t,p) = y_2(t,q)\quad \implies \quad p= q . \end$$
Simply put, we say that the within-host model Eq. (1) is structurally identifiable if two identical observation are only possible for identical parameters. We perform the structural identifiability analysis via differential algebra approach. The first step in this approach is eliminating the unobserved state variables from the within-host model Eq. (1). The reason for eliminating the unobserved state variables is to obtain a system which only involves the observed states and model parameters. Since this is a complex procedure, we use DAISY32 and obtain the following system
$$\begin \begin&\displaystyle \fracy_1 - \displaystyle \frac\displaystyle \frac + \displaystyle \frac y_1^2 \beta _u + \displaystyle \frac y_1(c_u + \delta _u) - \left( \displaystyle \frac\right) ^2 (c_u + \delta _u) + \displaystyle \frac \displaystyle \frack_l \\& \quad + \displaystyle \frac y_1^2 \beta _u (c_u + \delta _u) +\displaystyle \frac y_2 \delta _u k_l - \displaystyle \fracy_1k_l - \displaystyle \frac y_1^2 \beta _u k_l - \displaystyle \fracy_1 \delta _u k_l + y_1^3 \beta _u c_u \delta _u - y_1^2 y_2 \beta _u \delta _u k_l = 0.&\end \end$$
(3)
and
$$\begin \begin&-\displaystyle \frac y_2^5 k_u - 4 \displaystyle \frac y_2^4 K k_u - 6 \displaystyle \frac y_2^3 K^2 k_u - 4 \displaystyle \frac y_2^2 K ^3 k_u -\displaystyle \frac y_2 K^4 k_u + \displaystyle \frac \displaystyle \frac y_2^4 k_u \\&\quad + 4 \displaystyle \frac \displaystyle \frac y_2^3 K k_u + 6 \displaystyle \frac \displaystyle \frac y_2^2 K^2 k_u + 4 \displaystyle \frac \displaystyle \frac y_2 K^3 k_u + \displaystyle \frac \displaystyle \frac K^4 k_u \\&\quad - \displaystyle \frac y_2^6 \beta _l k_u + \displaystyle \frac y_2^5 k_u ( - 4 \beta _l K - \delta _l) + 2 \displaystyle \frac y_2^4 K k_u ( - 3 \beta _l K - 2 \delta _l) + 2 \displaystyle \frac y_2^3 K^2 k_u ( - 2 \beta _l K - 3 \delta _l) \\&\quad + \displaystyle \frac y_2^2 K^3 k_u ( - \beta _l K - 4 \delta _l) - \displaystyle \frac y_2 \delta _l K^4 k_u + \displaystyle \frac y_2^5 + 4 \displaystyle \frac y_2^4 K + 6 \displaystyle \frac y_2^3 K^2 + 4 \displaystyle \frac y_2^2 K^3 \\&\quad + \displaystyle \frac y_2 K^4 - \displaystyle \frac \displaystyle \frac y_2^4 - 4 \displaystyle \frac \displaystyle \frac y_2^3 K - 6 \displaystyle \frac \displaystyle \frac y_2^2 K^2 - 4 \displaystyle \frac \displaystyle \frac y_2 K^3 - \displaystyle \frac \displaystyle \frac K^4 \\&\quad + \displaystyle \frac y_2^6 \beta _l + \displaystyle \frac y_2^5 (4 \beta _l K + \delta _l) + 2 \displaystyle \frac y_2^4 K (3 \beta _l K + 2 \delta _l) + \displaystyle \frac y_2^3 K (4 \beta _l K^2 + c_l + 6 \delta _l K) \\&\quad + \displaystyle \frac y_2^2 K^2 (\beta _l K^2 + 2 c_l + 4 \delta _l K) + \displaystyle \frac y_2 K^3 (c_l + \delta _l K) - \displaystyle \frac^2 y_2^4 \delta _l - 4 \displaystyle \frac^2 y_2^3 \delta _l K \\&\quad + 3 \displaystyle \frac^2 y_2^2 K ( - c_l - 2 \delta _l K) - 4 \displaystyle \frac^2 y_2 K^2 (c_l + \delta _l K) - \displaystyle \frac^2 K^3 (c_l + \delta _l K) + \displaystyle \frac y_1 y_2^4 \delta _l k_u \\&\quad + 4 \displaystyle \frac y_1 y_2^3 \delta _l K k_u + 6 \displaystyle \frac y_1 y_2^2 \delta _l K^2 k_u + 4 \displaystyle \frac y_1 y_2 \delta _l K^3 k_u + \displaystyle \frac y_1 \delta _l K^4 k_u \\&\quad + \displaystyle \frac y_2^6 \beta _l \delta _l + 4 \displaystyle \frac y_2^5 \beta _l \delta _l K + \displaystyle \frac y_2^4 (\beta _l c_l K + 6 \beta _l \delta _l K^2 - c_l \delta _l) + 2 \displaystyle \frac y_2^3 K (\beta _l c_l K + 2 \beta _l \delta _l K^2 - c_l \delta _l) \\&\quad + \displaystyle \frac y_2^2 K^2 (\beta _l c_l K + \beta _l \delta _l K^2 - c_l \delta _l) - y_1 y_2^6 \beta _l \delta _l k_u - 4 y_1 y_2^5 \beta _l \delta _l K k_u - 6 y_1 y_2^4 \beta _l \delta _l K^2 k_u \\&\quad - 4 y_1 y_2^3 \beta _l \delta _l K^3 k_u - y_1 y_2^2 \beta _l \delta _l K^4 k_u + y_2^6 \beta _l c_l \delta _l + 3 y_2^5 \beta _l c_l \delta _l K + 3 y_2^4 \beta _l c_l \delta _l K^2 + y_2^3 \beta _l c_l \delta _l K^3 =&0.\\ \end \end$$
(4)
Equations (3) and (4) are called input–output equations of within-host model Eq. (1), which are differential polynomials involving the observed state variables \(y_1=V_u(t)\) and \(y_2=V_l(t)\) and the within-host model parameters. Note that solving input–output equations Eqs. (3) and (4) is equivalent to solving the within-host model Eq. (1) for the state variables \(V_u(t)\) and \(V_l(t)\). For identifiability analysis, it is crucial that the input-output equations are monic, i.e. the leading coefficient is 1. It is clear that the input–output equation Eq. (3) is monic, and the input–output equation Eq. (4) can be made monic by dividing the coefficients with the coefficient of the leading term, which is \(k_u\). As a result, the definition of the structural identifiability within differential algebra approach which involves input–output equations takes the following form27,28,29,30.
Definition 2
Let \(c(p)\) denote the coefficients of the input-output equations, Eqs. (3) and (4), where \(p\) is the vector of model parameters. We say that the within-host model Eq. (1) is structured to reveal its parameters from the observations if and only if
$$\begin c(p) = c(q) \quad \implies \quad p= q. \end$$
Suppose \(p = \\) and \(q = \}_u,}_u,}_u,}_u,}_l,}_l, }_l,}_l,}_l,},}_u\}\) are two parameter sets of the within-host model which produced the same observations. This can only happen if the coefficients of the input-output Eqs. (3) and (4) are the same. Hence, if \(c(p )\) denote the coefficients of the corresponding monic polynomial of input–output equations, we solve \(c(p ) = c(q)\) to obtain
$$\begin \beta _u = }_u,\, \delta _u = }_u,\, c_u = }_u,\, k_u = }_u, \,\beta _l = }_l,\, \delta _l = }_l,\, c_l = }_l,\, K = },\, k_l = }_l. \end$$
(5)
The solution set (5) means that the parameters, \(\beta _u, \delta _u, c_u, k_u, \beta _l,\delta _l,c_l,K\) and \(k_l\) can be identified uniquely. However, parameters \(p_u\) and \(p_l\) both disappear from the input–output Eqs. (3) and (4). It is easier to see the reason behind this by scaling the unobserved state variables of the within-host model Eq. (1) with a postive scalar \(\sigma >0\). Hence, \((\sigma T_u,\sigma I_u, V_u, \sigma T_l,\sigma I_l,V_l) = (}_u,}_u, V_u, }_l,}_l,V_l)\) will solve the following system
$$\begin \begin \frac}_u} =&-\beta _u }_uV_u,\\ \frac}_u} =\,&\beta _u }_uV_u,- \delta _u }_u, \\ \frac =\,&}_u }_u - c_uV_u + k_l V_l, \\ \frac}_l} =&-\beta _l }_l V_l,\\ \frac}_l} =\,&\beta _l}_l V_l- \delta _l }_l, \\ \frac =\,&}_l }_l - c_l \fracV_l + k_u V_u,\\ \end \end$$
(6)
where \(}_u = \frac\) and \(}_l = \frac.\) Since \(\sigma > 0\) was arbitrary and the observations do not give information about the scaling parameter \(\sigma\), the parameters \(p_u\) and \(p_l\) can not be identified from the viral load in the URT and LRT tracts. We conclude that the within-host model Eq. (1) is not identifiable. We summarize the structural identifiability result in the following Proposition 1.
Proposition 1
The within-host model Eq. (1) is not structured to reveal its parameters from the observations of viral load in upper and lower respiratory tracts. The parameters \(p_u\) and \(p_l\) are not identifiable and only the parameters \(\beta _u,\delta _u,c_u,k_u,\beta _l,\delta _l,c_l,K,k_l\) can be identified.
To obtain a structurally identifiable model from the \(V_u\) and \(V_l\) observations, we scale the unobserved state variables with \(}_u = p_u T_u, \,}_u = p_u I_u, \, }_l = p_l T_l, \, }_l = p_l I_l\) and obtain the following scaled within-host model
$$\begin \begin \frac}_u} =&-\beta _u }_u V_u,\\ \frac}_u} =\,&\beta _u }_u V_u,- \delta _u }_u, \\ \frac =\,&}_u - c_uV_u + k_l V_l, \\ \frac}_l} =&-\beta _l }_l V_l,\\ \frac}_l} =\,&\beta _l }_l V_l- \delta _l }_l, \\ \frac =\,&}_l - c_l \fracV_l + k_u V_u.\\ \end \end$$
(7)
Proposition 2
The scaled within-host model Eq. (7) is structured to reveal its parameters from the observations of viral load in upper and lower respiratory tracts. All the parameters
$$\begin \beta _u,\delta _u,c_u,k_u,\beta _l,\delta _l,c_l,K,k_l \end$$
can be identified, hence the within-host model Eq. (7) is globally identifiable.
Data fitting
Parameter values
We assume that the upper respiratory tract susceptible population is \(T_0^u=4\times 10^8\) epithelial cells, as in influenza studies23. This estimate was obtained by assuming a URT surface in adults of 160 cm\(^2\)33 and an epithelial cell’s surface area of \(2 \times 10^ - 4 \times 10^\) m\(^2\)34. We use a similar method to estimate the target cell population in the LRT. The lung’s surface area of 70 m\(^2\) (with range between 35 m\(^2\) and 180 m\(^2\))35 is composed of gas exchange regions (aveoli), and of conducting airways (trachea, bronchi, bronchioles). Since the gas exchange region is affected by SARS-Cov-2 only in severe cases20 we ignore it, and restrict the LRT compartment to the conducting airways whose surface area is \(2471 \pm 320\) cm\(^2\)36. Therefore, we obtain an initial epithelial cell target population in the LRT of \(T_l^0 = 6.25 \times 10^\) epithelial cells. If we assume that viral production rates are \(p_u=50\) and \(p_l=32\) per day then, after scaling, we have initial target cell populations in the URT and LRT of \(}_^0 = 2 \times 10^\) epithelial cells and \(}_l^0 = 2 \times 10^\) epithelial cells. The other initial conditions are unaffected by scaling and are set at \(}_u^0= }_L^0=0\), \(}_u^0= 0.1\) and \(}_L^0=0\), where the virus inoculum of \(}_u^0 = 0.1\) cp/ml is set below the reported limit of quantification of \(10^2\) cp/ml18. Lastly, the incubation periods were estimated in Ref.19 and are listed in Table 1.
Bayesian parameter estimation
During the data collection process, observations are perturbed with noise. Hence, the URT and LRT viral load deviates from the smooth trajectory of the observations \(y_1(t)\) and \(y_2(t)\) at measurement times. We represent measurement error using the following statistical model
$$\begin \begin&V_u^(t_i) = y_1(t_i, }) +\epsilon _i\quad i=1,2,\ldots ,n_u;\\&V_l^(t_j) = y_2(t_j, }) +\epsilon _j\quad j=1,2,\ldots ,n_l; \end \end$$
(8)
where \(}}\) is the true parameter vector assumed to generate the data, and the random variables \(\epsilon _i\) and \(\epsilon _j\) are assumed to be Gaussian with mean zero and standard deviation \(\sigma\).
We use Bayesian inference and Markov Chain Monte Carlo (MCMC) to determine the remaining nine parameters of the model Eq. (7)
Bayesian inference treats model parameters as random variables and seeks to determine the parameters’ posterior distribution, where the term “posterior” refers to data-informed distributions. The posterior densities are determined using Bayes’ theorem, which defines them as the normalised product of the prior density and the likelihood. Let \(\pi (p| })\) denote the probability distribution of the parameter \(p\) given the data \(} = \Big (V_u(t_i), V_l(t_j)\Big )\), then the Bayes theorem states that
$$\begin \pi (p| }) = \displaystyle \frac} |p) \pi (p)}})}, \end$$
where \(\pi (p)\) is the prior parameter distribution and \(\pi (})\) is a constant which is usually considered to be a normalization constant so that the posterior distribution is indeed a probability density function (pdf), i.e. its integral equals to 1. The likelihood function \(\pi (} |p)\) gives the probability of observing the measurements \(}\) given that the parameter values is \(p\). In terms of the within-host model Eq. (7) and the laboratory data Eq. (8), the likelihood function \(\pi (} |p)\) takes the following form
$$\begin \begin \pi (} |p) =&\displaystyle \prod _^ \displaystyle \frac\pi \sigma ^2} e^\left( \log _(V_u(t_i))-\log _(V_u^(t_i)\right) ^2} \\&\quad \times \displaystyle \prod _^ \displaystyle \frac\pi \sigma ^2} e^\left( \log _(V_l(t_j))-\log _(V_l^(t_j)\right) ^2}. \end \end$$
(9)
The ultimate goal is to determine the posterior distributions of the parameters in the light of laboratory data. To approximate the posterior distributions, we use the MCMC method introduced in Refs.37,38. MCMC methods generate a sequence of random samples \(p_1,\ p_2,\ldots , p_N\) whose distribution asymptotically approaches the posterior distribution for size N. The random walk Metropolis algorithm is one of the most extensively used MCMC algorithms. The Metropolis algorithm starts at position \(p_i\), then the Markov chain generates a candidate parameter value \(p_*\) from the proposal distribution, and the algorithm accepts or rejects the proposed value based on probability \(\alpha\) given by
$$\begin \alpha = \min \left( \displaystyle 1, \frac\right) . \end$$
As with the Metropolis algorithm, the essential feature of MCMC approaches is the formulation of a proposal distribution and an accept–reject criteria. In this paper, we employ the Delayed Rejection Adaptive Metropolis, (DRAM37) and use the MATLAB toolbox provided by the same authors39. In comparison to other Metropolis algorithms, the Markov chain constructed with DRAM is robust and converges rapidly (see Fig. 2).
The Markov chain of the within-host model Eq. (7)’s parameters obtained when the model is fitted to the population data. Every 1000th point of \(10^6\) iterations are shown. The black line shows the mean of the chain.
The two patch within-host model Eq. (7) is novel, hence we do not have any prior information regarding model parameters. We determine the prior distributions by fitting the structurally identifiable within-host model Eq. (7) to patient A’s data and to the population data (all nine patients). The prior distributions \(\pi (p)\) are then defined as a normal distribution with a mean equal to the fitted value and variance equal to \(\sigma ^2\), \(\pi (p) \sim N (\mu , \sigma ).\) Table 2 shows the obtained prior distribution of each parameter for patient A and population data, together with the lower and upper bounds of the prior \(\pi (p)\).